By Gordon Rugg and Sue Gerrard
This is the third post in a short series on semi-tacit and tacit knowledge. The first article gave an overview of the topic, structured round a framework of what people do, don’t, can’t or won’t tell you. The second focused on the various types of do (explicit) and don’t (semi-tacit) knowledge. Here, we look at can’t (strictly tacit) and won’t knowledge.
The issues involved are summed up in the diagram below.
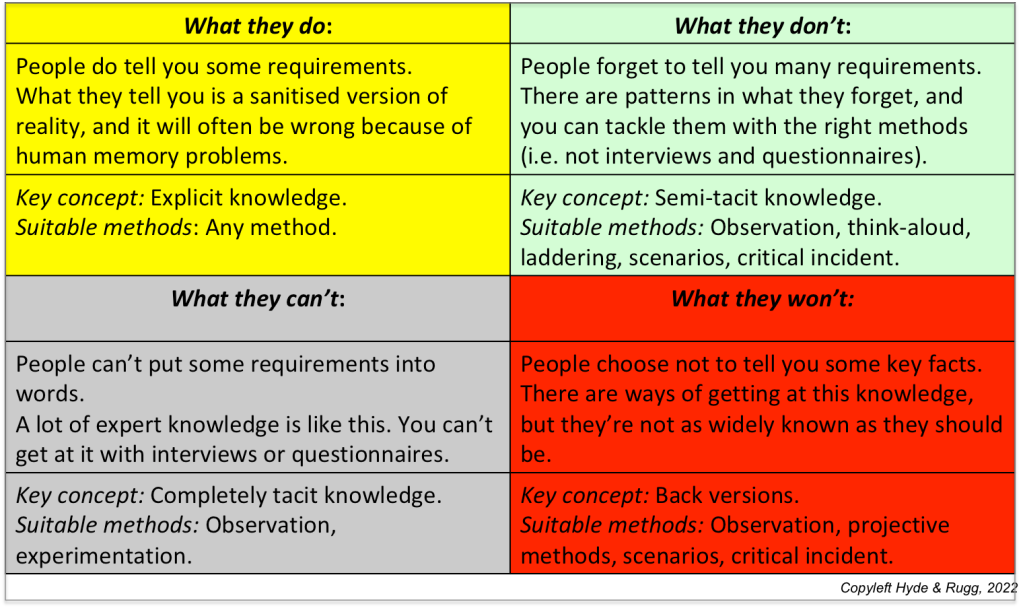
Can’t: Strictly tacit knowledge
The common factor in don’t knowledge is that the person involved can access the knowledge in some contexts, but not in all contexts. The common factor in can’t knowledge is that the person involved can’t immediately – if at all – access that knowledge explicitly in any context. They may be able to use the knowledge, and often use it at a very high level of skill, but they won’t be able to tell you what the knowledge is. They may refer to it using terms such as gut feel or intuition or muscle memory.
There are several types of can’t knowledge, each with very different implications. A common factor across these types is that the brain handles most tasks via massively parallel associative networks, rather than via step-by-step serial reasoning of the type used in classical formal logic. The parallel associative approach is much faster than the serial approach, but its contents and mechanisms are typically a “black box” inaccessible even to the person using it.
Compiled skills are skills that the person learned via explicit do knowledge, but they can now use without thinking, and would have to think hard to describe verbally. A classic example is changing gear when driving a vehicle; this skill starts as explicit do knowledge, but is increasingly handled by compiled can’t knowledge as the driver becomes more expert. Compiled skills are typically faster and more fluent than explicit do skills, as in the case of expert drivers. As a rule of thumb, if a person can carry out a task while maintaining a conversation, without a significant dip in performance, then they are probably using compiled skills.
Compiled skills typically require repeated practice over time, in the order of weeks or longer. They probably involve myelination of the neural pathways used in the task, making those pathways swifter and less susceptible to disruption. The nature of these pathways means that compiled skills are liable to strong but wrong errors, e.g. following your usual route to work and forgetting to detour to pick up a prescription from the pharmacy. Compiled skills often involve physical actions, which are sometimes referred to as muscle memory, but may also include sequences of mental actions.
Implicit learning involves someone gradually learning an association without being able to articulate that knowledge. This may take various forms. An early example in the research literature involved people in the poultry industry learning to tell whether a newly hatched chick is male or female without using surgical inspection. They were unable to explain how they knew whether the chick was male or female, but their judgments were correct almost all the time. Another example involved people predicting outputs from a simulated factory, again performing well, but without being able to articulate how their knowledge worked.
Implicit learning typically requires very large numbers of training examples, in the order of thousands or tens of thousands, and a significant training time, in the order of days or weeks.
Incidental learning is different from the processes above in that it can occur after a single example. It is also different in that it can occur explicitly, semi-tacitly, or tacitly. It involves learning something unintentionally while paying attention to something else.
For instance, a novice may see an expert perform a task in a particular way, and then perform the task in the same way as the expert. Sometimes this will happen explicitly, often accompanied by an “aha” moment; sometimes it will happen completely tacitly, with the beginner utterly unaware of where they learned to do the task in that way. The traditional apprentice task of sweeping the workplace floor provides extended opportunities for incidental learning.
Pattern matching is facilitated by associative networks; it’s the mechanism that most humans use to recognise familiar faces, or to tell that something is a pencil as opposed to a pen. It may occur at the level of surface features, or at the level of deep structure. For example, recognising a particular face involves surface feature pattern matching, whereas recognising that two different problems have the same underlying cause involves deep structure pattern matching.
Pattern matching is massively parallel, involving the cognitive processing of large numbers of items simultaneously. Face recognition, for instance, involves processing information about the component facial features as well as overall shape. This is very different from serial processing, which entails processing information one step at a time.
Parallel processing is much faster than serial processing, and can handle problems such as three dimensional pattern recognition that serial processing finds difficult or impossible. However, it is prone to errors that are the mirror image of its strengths, typically involving premature closure as soon as it finds a possible pattern match. This first match may be completely spurious, as in pareidolia. We’ll expand on parallel and sequential processing in the next post.
Won’t:
There are various reasons for people being unwilling to divulge information or knowledge, ranging from professional confidentiality to taboo topics and to criminal liability.
Projective methods can get at some won’t knowledge. These involve asking the person what someone else would do; for instance, What would a bad student do in this situation? This approach can be used with most elicitation methods; for example, you can ask someone to perform a card sort as if they were a dishonest customer.
Projective methods are useful for generating insights into what might be going on, but they need to be used with caution. One risk is that they will elicit stereotypes rather than responses based on direct factual experience. For instance, a response suggesting that members of a particular group will cheat in a test may well be a stereotype; however, a response saying that a bad student would cheat in a specific way might well indicate a mechanism for cheating that the examiners were previously unaware of.
Indirect observation involves observing proxies for a behaviour, as opposed to observing a behaviour itself. For instance, sociologists have used the dates of recorded marriages and first births as a way of indirectly observing the incidence of premarital sex in past times.
Conclusions and further thoughts
In this post, we’ve focused on the implications of can’t (i.e. strictly tacit) and won’t knowledge for eliciting people’s knowledge. There are also significant implications for other areas, such as:
- How best to represent the different types of can’t and won’t knowledge (e.g. verbally, or schematically, or via images, or via senses other than visual)
- The interaction between these knowledge types and types of human error; for instance, compiled skills are particularly liable to causing strong but wrong errors
- Mapping these knowledge types onto techniques for teaching and learning; for instance, compiled skills will typically require at least two weeks of practice to become properly compiled, whereas incidental learning may occur within seconds.
We will address these issues in later posts. We hope you have found this one interesting and useful.
Links and references
Card sorts: A short tutorial, and an in-depth tutorial.
Laddering: A short tutorial, and a longer tutorial (PDF download).
Observation:
- An overview
- The STROBE method for making inferences about a working environment from observation.
- Identifying issues with the built environment, part 1
- Identifying issues with the built environment, part 2
Reports: An overview that includes think-aloud, critical incident technique, hard case technique, and scenarios.
Content analysis:
Associative networks:
Requirements:
Aesthetics and design:
Reference to original article:
The Maiden & Rugg ACRE reference is: Maiden, N.A.M. & Rugg, G. (1996). ACRE: a framework for acquisition of requirements. Software Engineering Journal, 11(3) pp. 183-192.
Copyleft: you’re welcome to use the image in this article for any non-commercial purpose, including lectures, as long as you retain the coplyleft statement within the image.